Please join us Monday, September 19, at 4:30 p.m. in the newly renovated Strange Lounge of Main Hall for the return of the Strange Philosophy Thing.
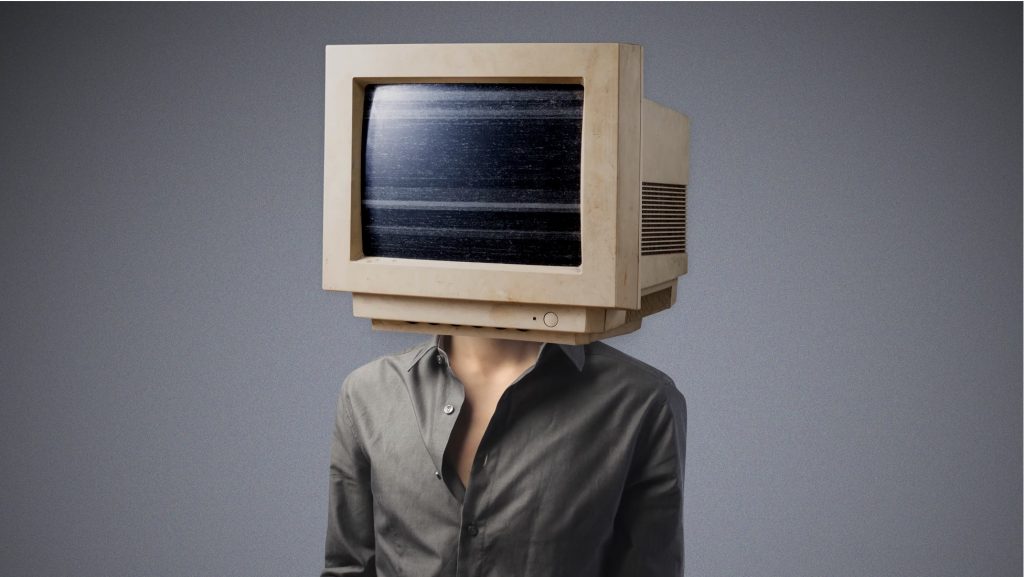
Language models are computational systems designed to generate text by, in effect, predicting the next word in a sentence. Think of the text-completion function on your cell phone. Large language models (LLMs) are language models of staggering complexity and capacity. Consider, for example, OpenAI’s GPT-3, which Tamkin and Ganguli note, “has 175 billion parameters and was trained on 570 gigabytes of text”. This computational might allows GPT-3 an as yet unknown number of capabilities—unknown, because they are uncovered when users type requests for it to do things. Among its known capabilities are summarizing lengthy blocks of text, designing an advertisement based on a description of the product, writing code to accomplish a desired program effect, participating in a text chat, and writing a term paper or a horror story. Early users report that GPT-3’s results are passably human. And LLM’s are only destined to improve. Indeed, artificial intelligence researchers expect LLMs to serve a central role in attempts to create artificial general intelligence. Our discussion on Monday (9/19) will focus on two aspects of this research:
- Are LLMs genuinely intelligent?
The issues we will discuss this week are alluded to in John Symon’s recent article in the Return. To frame our discussion about the general intelligence of LLMs, we might consider the following thought experiment, as discussed by Symons:
Alan Turing had originally conceived of a text-based imitation game as a way of thinking about our criteria for assigning intelligence to candidate machines. If something can pass what we now call the Turing Test; if it can consistently and sustainably convince us that we are texting with an intelligent being, then we have no good reason to deny that it counts as intelligent. It shouldn’t matter that it doesn’t have a body like ours or that it wasn’t born of a human mother. If it passes, it is entitled to the kind of value and moral consideration that we would assign to any other intelligent being.
LLMs either already do (or, it is plausible to suppose, they will soon) pass the Turing Test. Are we comfortable with the conclusions Symons derives from this fact—that LLMs “count as intelligent” and are “entitled to…moral consideration”? Perhaps we should rather reappraise the Turing Test itself, as a recent computer science paper suggests we should do. If LLMs can pass the test by merely reflecting the intelligence of the tester, then perhaps the true test is to have LLMs converse with one another and see if we judge them as intelligent from outside of the conversation.
- Are LLMs making us less intelligent?
A second—and the more important—theme in Symons article is the role that LLMs might play in making us less intelligent. Symons’ claims here are built on his own observations about LLMs. As he writes:
I tried giving some of these systems standard topics that one might assign in an introductory ethics course and the results were similar to the kind of work that I would expect from first-year college students. Written in grammatical English, with (mostly) appropriate word-choice and some convincing development of arguments. Generally, the system accurately represented the philosophical positions under consideration. What it said about Kant’s categorical imperative or Mill’s utilitarianism, for example, was accurate. And a discussion of weaknesses in Rawlsian liberalism generated by GPT-3 was stunningly good. Running a small sample of the outputs through plagiarism detection software produced no red flags for me.
Symons notes—rightly it seems—that as the technology progresses students will be tempted to use LLMs to turn in assignments without any effort. Instructors, including college professors, will be unable to detect that LLMs, rather than students, generated fraudulent assignments. But, while this might seem a convenient way to acquire a degree, Symons argues that it would undermine the worth of the education that the degree is meant to convey. For—or at least as Symons maintains—learning to write is learning to think, and deep thought is possible only when scaffolded by organized prose. Students completing writing assignments, then, are learning to think, and when they rely on LLMs (or other means) to avoid writting assignments, they are cheating themselves of future competent thought. Focusing on the discipline of philosophy in particular, Symons writes:
…most contemporary philosophers aim to help their students to learn the craft of producing thoughtful and rationally persuasive essays. In some sense, the ultimate goal of the creators of LLMs is to imitate someone who has mastered this craft. Like most of my colleagues who teach in the humanities, philosophers are generally convinced that writing and thinking are connected. In some sense, the creators of GPT-3 share that view. However, the first difference is that most teachers would not regard the student in their classroom as a very large weighted network whose nodes are pieces of text. Instead, the student is regarded as using the text as a vehicle for articulating and testing their ideas. Students are not being trained to produce passable text as an output. Instead, the process of writing is intended to be an aid to thinking. We teach students to write because unaided thinking is limited and writing is a way of educating a student in the inner conversation that is the heart of thoughtful reflection.
These passages might constitute a jumping off point for our second topic of discussion. Ancillary questions here might include :1) What is the purpose of writing assignments in college? 2) To what extent is complex thought possible without language? 3) How can we design education in such a way as to produce the best possible thinkers and writers?
I look forward to seeing you all on Monday at 4:30 for a fun, informal discussion, open to everyone! Philosophy professors and snacks provided.